How in silico methods accelerate innovations in biotechnology and pharmaceuticals
Revolutionizing pharma: In silico methods in startups boost drug development, merging tech and biology for rapid innovation.
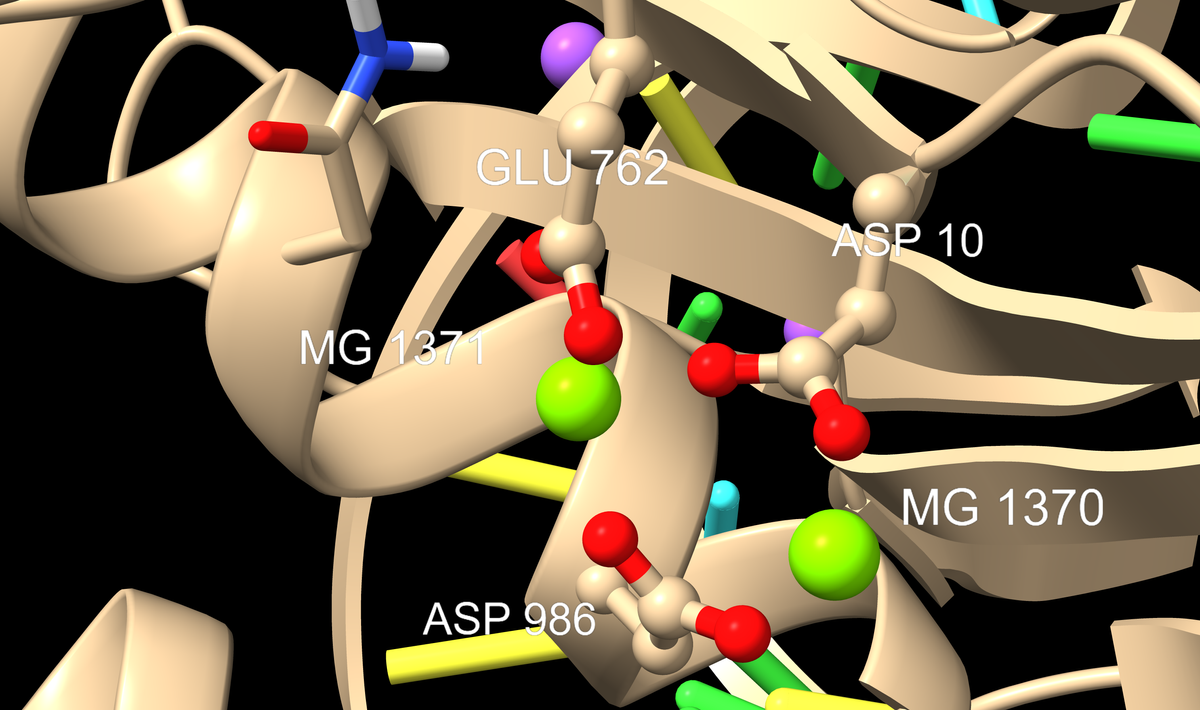
A significant number of scientific and technological startups have seen development in recent years. What was once possible only at the state level is gradually shifting into the hands of enthusiasts. Scientific and technological startups have some advantages over government research centers: less bureaucracy and a higher proportion of motivated employees. However, startup founders do not always have a strategic development plan. This is due to the complexity of scientific research, which is an integral part of a scientific and technological startup. Many leaders of such projects may face a crisis of ideas and the dilemma of choosing the further modernization of the product after a successful start. The development of any product, from drugs to protein vaccines, is a very complex and costly process. We can observe this in the development of pharmaceuticals: in recent decades, pharmacies have seen a large number of drugs with good therapeutic effects and tolerability. This is the result of both the overall development of pharmacology and the introduction of in silico methods in particular. The screening and selection stage from thousands of candidate compounds cannot be carried out without in silico methods. They are responsible for the exponential growth in the number of discovered compounds. This acceleration is due to the fact that in silico methods allow for the virtual reproduction of the key chemical properties of a substance based on its atomic structure. It is these methods that will be discussed now.
The leader of a scientific and technological startup often has to make a difficult decision during research planning. Any product needs development and modernization, and the paths of development and modernization are often not obvious. Experiments need to be planned, the results of which are unpredictable, and if you move blindly, you can spend time and money on reagents without definite success. In silico methods are needed to look a few steps ahead and eliminate obviously futile research paths or discover new ones that were not obvious before.
For convenience, let's consider several key in silicomethods and their application in a hypothetical situation.
1. MD Simulation
Let's assume we are a biotechnological startup working on developing enzymes for making starter cultures. Modern biotechnology has technologies for directed mutagenesis, meaning it's hypothetically possible to obtain a protein with any sequence. Given that enzymes can have several hundred amino acids, finding a more active enzyme through brute-force experimentation would be very expensive. Without in silico methods, the startup would progress very slowly, collecting data on point mutations from available publications and conducting experiments to obtain modified enzymes. Most of these experiments would likely fail, as mutations are often non-additive, and if two amino acid substitutions each lead to an improvement in enzyme properties, applying them simultaneously may have the opposite effect.
This is where Molecular Dynamics (MD) simulation comes in handy. With the atomic structure of the enzyme as input (often key structures are available in the rcsb.org database), we can generate several mutants by simply replacing target amino acids in a 3D molecular editor. It's preferable to include a couple of mutants that have been experimentally validated for understanding their properties. Then, the simulation process is initiated on a computer, resulting in trajectories of conformational changes in the mutant enzyme molecules relative to the native one. From these trajectories, numerous important conclusions can be drawn, such as which mutants have a more rigid or flexible conformation, which ones tend to compact or decompact, etc. Subsequently, by experimenting with a few objects, e.g., those with the most rigid and most labile conformations, one can understand how the freedom of conformational transitions is related to enzyme activity. After this, the second phase can begin - selecting in silico mutations that would enhance the desired aspect of conformational transitions. Instead of a blind search over a long distance, we achieve cost savings on reagents, work, and time through virtual analysis of the enzyme's protein structure.
2. QM/MM Studies
Now, let's imagine that we have launched a pharmaceutical compound on the market. Every drug has several generations, with each subsequent generation supposed to be more effective and safer than the previous one. How do we search for a new substance? Before the advent of in silico methods, there was a whole branch of chemistry that has fortunately become history - combinatorial chemistry. Researchers would test dozens of structures, each with slight differences, on a large number of experimental animals. Positive effects were rarely observed in any group. More often than not, the efforts of synthetic chemists and experimenters were unrewarded. Thanks to the QM/MM approach, we can model a system with a receptor protein and our drug candidate. After preliminary MD calculations or docking studies, we can obtain the geometry of the complex between our substance and the receptor. To assess how well the substance binds to the receptor, we need to calculate, within the framework of QM theory, the interaction energy between the drug and the protein. We can then modify the drug's structure based on our ideas, intuition, insights, etc., and compare the energies of the resulting complexes with a reference. The modification with higher binding energy becomes a potential candidate for the next generation of investigated drugs. It's advisable to gather several such candidates since unforeseen side effects may arise in subsequent experiments, making them challenging to predict. If the original substance has side effects, we can model its interaction with another receptor responsible for the side effect, but this time aiming for a structure with lower binding energy. This broadly outlines how in silicomethods can significantly narrow down the search and save time and money on experiment optimization.
A couple of words for conclusion...
In this article, we briefly touched upon in silico methods and their application. In silico is a separate branch of scientific research with a rich arsenal of tools. Essentially, the combination of different methods allows for modeling any task and solving it. LambasLab's goal is to assist scientific and technological startups in using in silico methods to develop their products and enrich humanity with new high-quality products.